Ghazal Fazelnia Designed Risk Assessment Model While Interning at J.P. Morgan
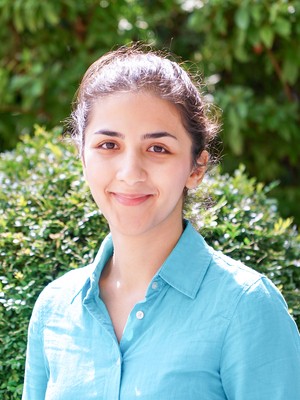
Electrical Engineering PhD Student Ghazal Fazelnia, did a summer internship at J.P. Morgan, where she designed a model to predict the risks associated with large bank loans.
Fazelnia was part of a team that assisted the company’s investment banking division, which issues loans to large corporations. Her model was a huge success - it was 50 percent more effective in assessing risk than previous models used by J.P. Morgan. The only intern on the team, she used machine learning and other data techniques she learned at Data Science Institute (DSI) to create the model.
Fazelnia, a doctoral student at Columbia Engineering, excels at research. In 2014, she was one of 10 students in the United States and Canada to win the Microsoft Research Graduate Women’s Scholarship, given to outstanding women in the second year of their graduate studies. She was born in Iran, where she studied electrical engineering at Sharif University of Technology and came to Columbia to do a master’s and Ph.D. in electrical engineering. In the course of her doctoral studies, though, she took an abiding interest in optimization theory, statistics and machine learning and so became a data scientist.
Growing up in Iran, Fazelnia was fortunate to have had a brilliant role model in Maryam Mirzakhani – the only woman in the world to win the Fields Prize for mathematics. Mirzakhani died earlier this year from breast cancer at 40, but Fazelnia will forever seek motivation from her brilliance.
In this interview, Fazelnia discusses her internship at J.P. Morgan and her passion for using data science to solve complex problems.
________________
Can you talk about the success of the project you did for your internship?
Senior managers at J.P. Morgan were really happy with our model. In terms of assessing risk, they said it was a huge improvement upon the models they previously used. They said that the future success of the company depended upon using the new resources in machine learning. The company has a machine-learning research group that develops machine learning algorithms for financial applications, and the managers know that the group must grow. Even the CEO talked publicly about the importance of machine learning to the future success of J.P. Morgan.
What data science tools did you use during your internship?
I developed machine learning and data science techniques to evaluate market risk. Our model evaluated all the variables, macro and micro, related to financial risk. In our model, I used well-known algorithms in machine learning such as random forest and K nearest neighbors as well as more technical probabilistic Bayesian models. The market is affected by many internal and external factors. Our goal was to design machinery to systematically pick out the best factors in describing the market, tune the model parameters with the observed data, and then forecast future outcomes. We needed to keep the algorithms simple, robust under so called “noise in data” as well as scalable for big datasets.
Why did you apply for the J.P. Morgan internship?
My primary reason for applying was to see what types of financial data financial companies use; what challenges they face in analyzing their data; and to explore what machine learning has to offer to tackle those challenges.
I’ve worked in the data field for a while, and I have seen datasets from a variety of fields, such as medical data, transportation, climate data and so on. I was wondering why we don’t often see financial data? After my internship, I realized that the nature of their financial data is similar to most of the datasets we commonly work with, and perhaps a better connection between academic research and financial markets is needed to explore all the possibilities.
Can you describe the research you’re doing for your dissertation?
I’m designing a probabilistic model to infer hidden themes and structures in data. For example, in looking at the gene expression of cancer patients, my model would show if the patient has a genetic structure related to a certain cancer, or how different cancer types might be related. Another application of my research is in designing models to infer the general topic or theme in data texts. I also work on methods for optimization problems to make them faster and scalable for machine- learning applications. Almost all problems end up being optimization problems, either minimizing error or maximizing the accuracy of prediction. Optimization is a huge part of machine learning and my goal is to work in this interdisciplinary area to improve the current state-of-the-art methods.
Who is your adviser?
I’m advised by Professor John Paisley, who is a great mentor. We think about problems together, and he’s actively involved in my research. He’s a leading expert in probabilistic models, machine learning techniques and inference, and his guidance, insight and support are absolutely invaluable to me.
Do doctoral students associated with the Data Science Institute have good resources for their research?
The doctoral students associated with DSI are lucky. We have access to great academic resources. We get to work with some of the greatest professors and researchers in the field as well as having access to various high-performance computing clusters. That, along with the great seminars and on-campus events, make DSI a great place for everyone who aims to work in and make contributions to the burgeoning field of data science.
Why did you come to Columbia Engineering for your doctoral work?
I studied electrical engineering in Sharif University of Technology in Iran, which is one the most competitive undergraduate programs due to its strong resources. After coming to Columbia Engineering for a Ph.D. three summers ago, I won a lottery to take a summer class taught by Michael Jordan, one of the world’s experts in machine learning. He was super encouraging and I came out of the class thinking that machine learning was the future of technology. It was then that I realized machine learning is what I love to do.
You won the Microsoft Research Graduate Women’s Scholarship. What are your thoughts on gender inequity in the STEM fields?
I think we’ve been caught in gender-perpetuating cycle; there are more men in math and science, which in turn draws more male students to the STEM because they see it as a good field for men. Traditionally, girls haven’t seen many women in the field, so they don’t see themselves doing scientific work. But that is changing now. For instance, 45 percent of computer science majors at Columbia University this year are women. There are great opportunities and programs that encourage women to study and work in the STEM fields and help girls realize they are capable of doing anything they want in math, science and technology.
After you finish your degree, do you intend to work in academia or in industry?
I like doing research and I want to stay on the applied side – developing algorithms and designing models for analyzing data. I could work happily either for a large tech firm or for a university. I feel fortunate to be studying at a great university, which will hopefully allow me to use data science to make this world a better place for everyone to live.
Original article can be found here.