|
Summary

News story segmentation is
essential for video indexing, summarization, and intelligence exploitation.
In this paper, we applied and extended the Maximum Entropy statistical
model to system atically induce and eRectively fuse diverse features from
multiple levels and modalities, including visual, audio, and text, in
international broadcast news videos. We have included various features
such as motion, face, music/speech discrimination, speech rapidity, high-level
text segmentation information, prosody, etc., and some novel features
such as syllable cue terms and significant
pauses. The statistical fusion model is used to automatically discover
relevant features contributing to the detection of story boundaries. We
also introduced a novel feature wrapper to address heterogenous features
{ usually asynchronous, variant-timescale, and either discrete or continuous.
We demonstrated encouraging performance (F1 measures up to 0.76 in ABC
news, 0.73 in CNN news, and 0.90 in Mandarin news), presented how these
multi-level multi-modal features construct the probabilistic framework.
We further investigated and compared alternative statistical
approaches based on discriminative learning, i.e. Support Vector Machine
(SVM), and ensemble learning, i.e. Boosting. We evaluated the experiments
and analyze erroneous cases with Mandarin news and the context of TRECVID
video retrieval benchmarking event 2003 with around 120-hour CNN/ABC news
videos.
The following diagram illustrates
some of the features considered in discriminating a candidate point in
time to story boundary or not using the multi-modal features observed
in a surrounding window. Key issues include feature selection, spatio-temporal
model estimation, and handling of imbalanced data.
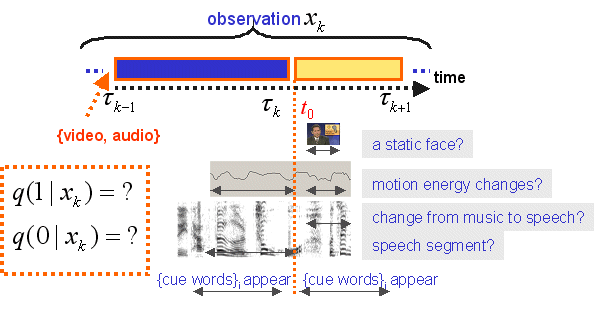
People

Publication

Arnon Amir, Marco Berg, Shih-Fu
Chang, Giridharan Iyengar, Ching-Yung Lin, Apostol (Paul) Natsev, Chalapathy
Neti, Harriet Nock, Milind Naphade, Winston Hsu, John R. Smith, Belle
Tseng, Yi Wu, Donqing Zhang, "IBM Research TRECVID-2003 Video Retrieval
System," Proceedings of TRECVID 2003 Workshop. (PDF)
Winston H.-M. Hsu and S.-F.
Chang, "Generative, Discriminative, and Ensemble Learning on Multi-modal
Perceptual Fusion toward News Video Story Segmentation," IEEE International
Conference on Multimedia and Expo, Taipei, Taiwan, June 27-30, 2004. (PDF)
Winston H.-M. Hsu, L. Kennedy,
C.-W. Huang, S.-F. Chang, C.-Y. Lin, G. Iyengar, "News Video Story
Segmentation using Fusion of Multi-Level Multi-modal Features in TRECVID
2003," International Conference on Acoustics, Speech, and Signal
Processing, Montreal, Canada, May 17-21, 2004. (PDF)
Winston H.-M. Hsu, S.-F. Chang,
C.-W. Huang, L. Kennedy, C.-Y. Lin, G. Iyengar, "Discovery and Fusion
of Salient Multi-modal Features towards News Story Segmentation,"
IS&T/SPIE Symposium on Electronic Imaging: Science and Technology
- SPIE Storage and Retrieval of Image/Video Database, San Jose, USA, January
18-22, 2004, invited paper. (PDF)
Chih-wei Huang, Winston Hsu,
Shih-Fu Chang, "Automatic Closed Caption Alignment Based on Speech
Recognition Transcripts," Columbia DVMM Technical Report 005,
2003. (PDF)
Winston H.-M. Hsu, Shih-Fu
Chang, "A Statistical
Framework for Fusing Mid-level Perceptual Features in News Story Segmentation,"
IEEE International Conference on Multimedia & Expo, 2003, invited
paper.(PS.GZ/PDF),
slides
Link


For problems or questions
regarding this web site contact The
Web Master.
Last updated: December 05, 2003.
|